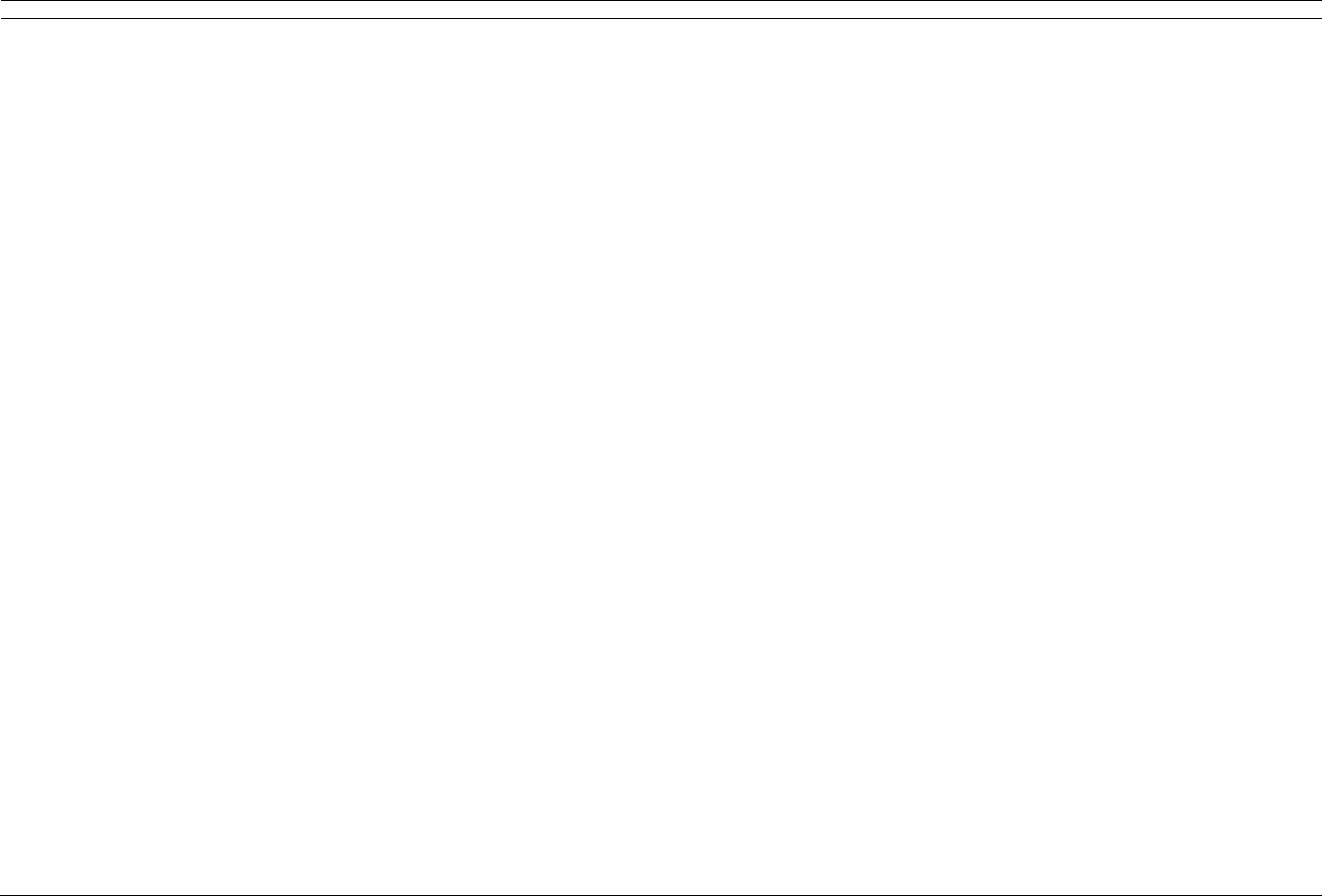
Online Appendix (Not For Publication)
Table A.2 - Cities in the Micro Price Data
Milton Keynes, United Kingdom
Sao Jose dos Campos, Brazil
Milwaukee, WI, United States
Phoenix, AZ, United States
Columbus, OH, United States
Minneapolis, MN, United States
Sarajevo, Bosnia And Herzegovina
Pittsburgh, PA, United States
Abu Dhabi, United Arab Emirates
Birmingham, United Kingdom
Guatemala City, Guatemala
Seattle, WA, United States
Guildford, United Kingdom
Port Elizabeth, South Africa
Portland, OR, United States
Dallas, TX, United States
Sharjah, United Arab Emirates
Boston, MA, United States
Las Vegas, NV, United States
Albuquerque, NM, United States
Leicester, United Kingdom
Hartford, CT, United States
Denver, CO, United States
Detroit, MI, United States
Ho Chi Minh City, Vietnam
Nashville, TN, United States
Spokane, WA, United States
Anchorage, AK, United States
Honolulu, HI, United States
Liverpool, United Kingdom
Washington, DC, United States
Houston, TX, United States
New Orleans, LA, United States
Raleigh, NC, United States
Huntsville, AL, United States
New York, NY, United States
Dubai, United Arab Emirates
Newcastle Upon Tyne, United Kingdom
West Palm Beach, FL, United States
Buffalo, NY, United States
Wichita, KS, United States
Asheville, NC, United States
Indianapolis, IN, United States
Los Angeles, CA, United States
Louisville, KY, United States
Atlanta, GA, United States
Richmond, VA, United States
Edinburgh, United Kingdom
Austin, TX, United States
Nottingham, United Kingdom
Cambridge, United Kingdom
Jacksonville, FL, United States
Roanoke, VA, United States
Rochester, NY, United States
Baltimore, MD, United States
Jeddah (Jiddah), Saudi Arabia
Oklahoma City, OK, United States
Fort Lauderdale, FL, United States
Madison, WI, United States
Fort Worth, TX, United States
Johannesburg, South Africa
Orlando, FL, United States
Sacramento, CA, United States
Banja Luka, Bosnia And Herzegovina
Saint Louis, MO, United States
Kansas City, MO, United States
Salt Lake City, UT, United States
Charlotte, NC, United States
Manchester, United Kingdom
Thiruvananthapuram, India
San Antonio, TX, United States
San Diego, CA, United States
Chicago, IL, United States
San Francisco, CA, United States
San Jose, CA, United States
Christchurch, New Zealand
Cincinnati, OH, United States
Memphis, TN, United States
Cleveland, OH, United States
San Salvador, El Salvador
Santa Barbara, CA, United States
Philadelphia, PA, United States
Santo Domingo, Dominican Republic
Tucson, AZ, United States